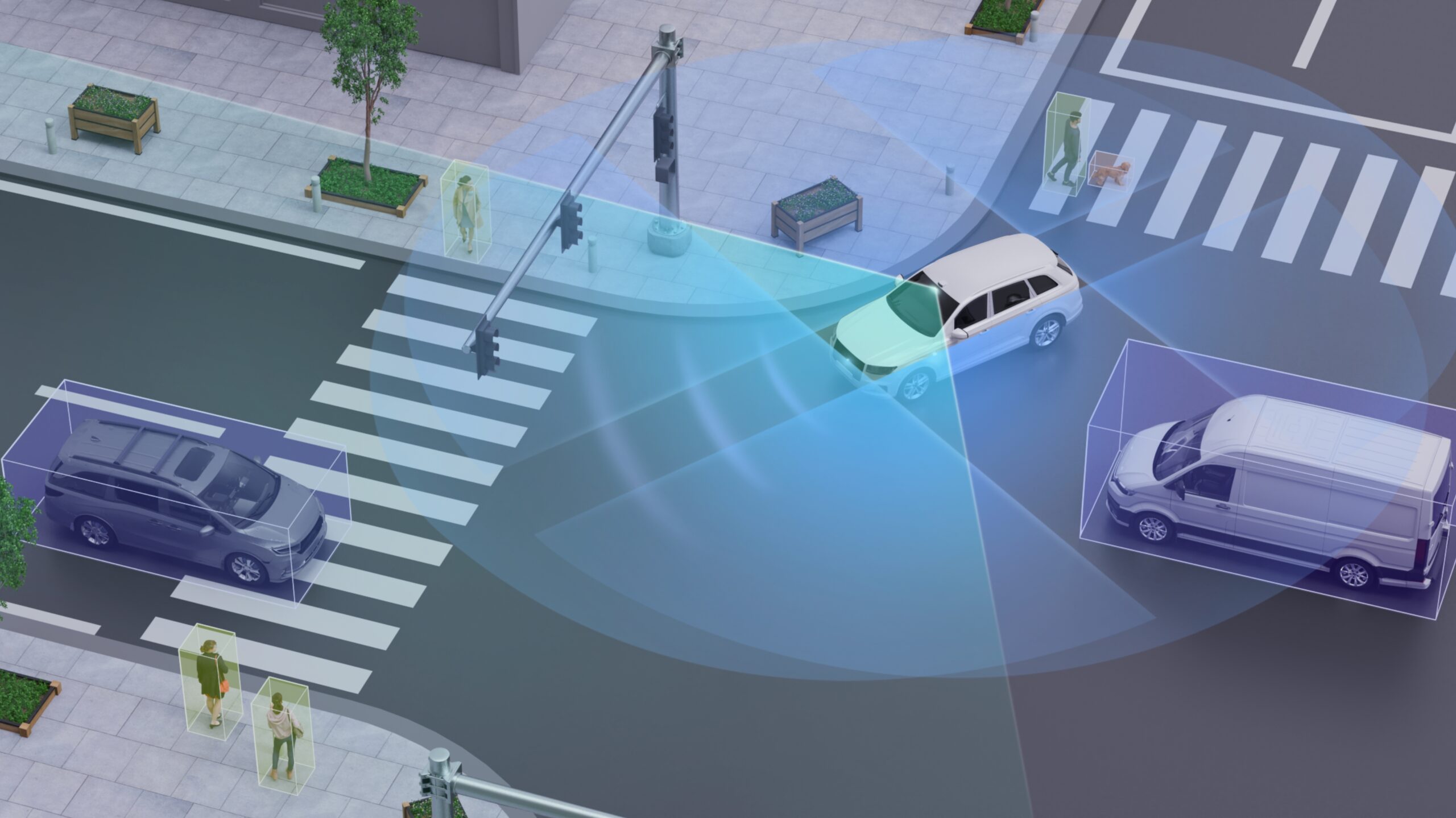
How LiDAR Is Unleashing the Full Potential of NOA Systems for Urban Driving
The popularization of urban driver assistance systems has sparked a surge of interest in vehicles equipped with lidar technology, which enables a comprehensive range of smart driving features. A notable example is Li Auto, which recently launched the trial for its new City NOA (Navigate on Autopilot) system in Shanghai and Beijing. The City NOA system will be followed by the nationwide rollout of the Commute NOA system during the second half of this year.
These two innovative NOA systems are featured in Li Auto’s Max models, which come with an onboard lidar sensor from Hesai. Hesai’s AT128 ultra-high resolution long-range lidar has 128 integrated laser channels, which can generate 1.53 million data points per second to map its surroundings in 3D. It also has an ultra-high resolution of 1200×128, and is capable of detecting obstacles up to 200 meters away.

Li Auto’s Max models are equipped with Hesai’s AT128 ultra-high resolution long-range lidar
Lidar-assisted NOA system offers safer and smarter driving in urban areas
During media tests in Beijing’s urban area, the Max model handled challenging traffic conditions with ease. Thanks to its outstanding performance, several media outlets praised the Max model for its “cutting-edge safety features” and “innovative City and Commute NOA systems, which will significantly increase the appeal of high-end vehicles”.
Based on a testing video released by the leading Chinese automotive media platform 42HOW, Li Auto’s NOA systems performed particularly well in the following scenarios:
Pedestrian in dark tunnel:

While in a dark tunnel, the vehicle accurately detected and avoided a pedestrian wearing black clothing.
Detecting a stationary vehicle from long range:

Vehicle detected a stationary truck from far away, providing sufficient time for lane change to avoid a collision.
Responding to complex urban traffic requires not only better sensors but a combination of different types of sensors to cover a multitude of driving scenarios.
To improve upon traditional BEV models that only rely on cameras, Li Auto adopted the advanced dynamic BEV data fusion model, combining other sensor inputs with lidar point cloud data. The fusion algorithm significantly improves the vehicle’s safety features in scenarios where camera-only solutions may fall short, such as complex urban environments, and glare from oncoming vehicles. In addition, it improves the system’s tracking accuracy and responsiveness, ensuring a smoother ride.

Li Auto’s dynamic BEV system combines image data from cameras with point cloud data from the onboard lidar sensor.[1]
The importance of accuracy in urban driver assistance systems
Urban driver assistance systems are called different names depending on the manufacturer, but their essential purpose is the same — they navigate the vehicle from one location to another through the often chaotic urban traffic. Under driver supervision, autonomous driving systems use a map to guide the vehicle to its destination, detecting lane markings, obstacles, and traffic lights in real time. This enables the vehicle to brake, change lanes, turn, and give way autonomously, offering a seamless driving experience.
The high degree of complexity and uncertainty is the greatest challenge in urban traffic. This includes anything from large numbers of pedestrians to unclear lane markings, complex junctions, unprotected left turns, traffic accidents, blind spots, and illegally parked vehicles. Nighttime conditions can be especially challenging due to reduced visibility and glare from oncoming vehicles.

Trial of Li Auto’s urban driver assistance system.
Lidar can provide accurate detection even in extreme scenarios
Cameras may struggle under dark environments, glare from oncoming vehicles, and sudden changes in brightness when entering and exiting tunnels. In contrast, lidar is not affected by any change in the surrounding brightness.
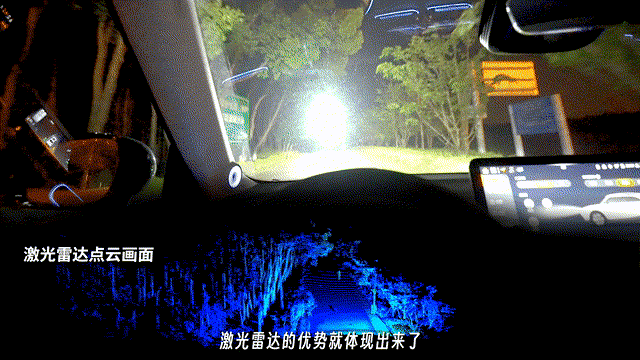
Lidar systems can accurately sense the surroundings and detect pedestrians
at long range, even when there is glare from oncoming vehicles.[2]

Nighttime performance of lidar
In addition, urban driver assistance systems must also be able to detect unidentifiable objects, such as overturned vehicles, rare animals, oddly shaped vehicles or atypical roadworks. The ability to detect and track obstacles is essential for the effective functioning of automated safety features such as AEB.
In lidar systems, objects appear as point cloud data, which the algorithm uses to calculate the physical space occupied and map a safe route to avoid a collision. Using point cloud data enables the vehicle to detect and avoid all types of obstacles, even if they cannot be categorized.

Lidar sensors can accurately detect unidentifiable obstacles.
Lidar-powered algorithms perform significantly better in accuracy metrics for driving safety
Sensing algorithms must be able to track and detect the position, dimensions and path of different types of objects. To assist with this process, the algorithm places objects into categories based on their characteristics and uses prior knowledge to predict their movement. For example, vehicles and pedestrians are two separate categories, as they move at very different speeds. The detection and tracking of objects are an essential prerequisite for City NOA systems and autonomous safety features such as AEB, AES and FCW.
One of the most common metrics for assessing the accuracy of object detection algorithms is called mAP (mean Average Precision).
According to test results from the industry-leading dataset nuScenes [6], lidar-powered algorithms have a significantly higher mAP than algorithms only relying on camera images. Algorithms that use a combination of lidar and camera technology have an average mAP of 73% (versus 57% for camera-only algorithms). The best performing algorithm had a mAP of 77%.

Source: nuScenes 2023 detection challenge.[3]
The addition of lidar technology also significantly improves the accuracy of object tracking, assessed with a metric called “Average Multi-Object Tracking Accuracy” (AMOTA), from 56% for camera-only solutions to 75% for solutions that use a combination of lidar and camera technology.

Source: nuScenes 2023 detection challenge.[4]
Based on the test results, a combination of lidar and camera offers the best detection and tracking accuracy, followed by lidar-only and camera-only solutions.

Lidar-assisted algorithms are able to accurately detect and track objects.
Accurate detection of road surface
To determine an object’s height above the ground and assess the need for avoidance, the algorithm must accurately detect the road surface. This capability is vital for various autonomous safety and driver assistance systems, including Autonomous Emergency Braking (AEB) and Adaptive Cruise Control (ACC).
Compared to other types of sensors, lidar systems exhibit superior accuracy when detecting road surfaces, ensuring a clear delineation between the road surface and objects high above the ground. Other sensors often struggle with low accuracy, limited range, and poor categorization, which may result in unnecessary braking, compromising comfort and potentially increasing the risk of accidents on highways. These challenges are amplified on hilly terrains or roads with sharp bends.
As the most accurate type of sensor for self-driving cars, lidar maps the surroundings in 3D, providing the algorithm with distance data with centimeter precision.

Lidar sensors can accurately detect the road surface.
Bumps and uneven road surfaces can also affect safety and comfort, especially at high speeds. Lidar-equipped vehicles are able to avoid these obstacles or reduce speed in advance in order to ensure a more comfortable ride.
More accurate lane and curb detection
Features such as lane keeping assist (LKA) and automatic lane change (ALC) rely on the vehicle’s ability to detect lane markings. This becomes particularly challenging on hilly terrain or roads with sharp bends, where camera-only systems may produce inaccurate results.
In addition to mapping the surroundings, lidar sensors can detect lane markings by measuring the reflectivity of the road surface.

Lidar sensors can reliably detect lane markings on slopes or bends.
Curbs are also an important part of the vehicle’s surroundings. However, camera-only systems often struggle to detect curbs, which are typically less than 20 centimeters above the road surface. In contrast, lidar systems are accurate to within 2 centimeters, understanding their position with much more precision.
Growing popularity of lidar-equipped vehicles
The accuracy of onboard sensors directly influences the performance of driver assistance systems. Lidar sensors are increasingly prevalent in all types of advanced driver assistance systems (ADAS) and autonomous safety features, including autonomous emergency braking (AEB), autonomous emergency steering (AES), forward collision warning (FCW), adaptive cruise control (ACC), lane keeping assist (LKA) and automatic lane change (ALC), providing drivers with a smarter driving experience. As ADAS technology continues to improve, more drivers are likely to opt for high-end models with lidar sensors.
【1】The footage is from Li Auto’s official WeChat channel
【2】The footage is from Leixing’s media channel
【3】Data is from:https://www.nuscenes.org/?externalData=all&mapData=all&modalities=Any, between 2023.1.1~2023.6.27